Pioneering the End-to-End AI Driving Model
In 2024, we introduced a groundbreaking approach to autonomous driving by replacing traditional rule-based, multi-head detection methods with an end-to-end AI network enhanced by Vision-Language Models (VLMs). This innovation mirrors human driving cognition by incorporating a “Thinking Fast and Slow” paradigm. Leveraging large VLMs, the system enhances image understanding and reasoning capabilities by applying common sense and logic, enabling it to analyze driving environments, make safe decisions in complex scenarios, and follow external human instructions—ultimately performing in a manner akin to human driving.
This approach represents a comprehensive, high-performance software stack designed to ensure the safe and efficient operation of self-driving vehicles, even in the most challenging environments.
Pioneering the End-to-End AI Driving Model
In 2024, we introduced a groundbreaking approach to autonomous driving by replacing traditional rule-based, multi-head detection methods with an end-to-end AI network enhanced by Vision-Language Models (VLMs). This innovation mirrors human driving cognition by incorporating a “Thinking Fast and Slow” paradigm. Leveraging large VLMs, the system enhances image understanding and reasoning capabilities by applying common sense and logic, enabling it to analyze driving environments, make safe decisions in complex scenarios, and follow external human instructions—ultimately performing in a manner akin to human driving.
This approach represents a comprehensive, high-performance software stack designed to ensure the safe and efficient operation of self-driving vehicles, even in the most challenging environments.
Advantages of the HOS2.0 Approach
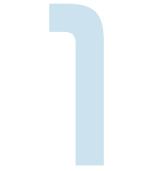
Driving Scene Understanding
By leveraging robust Vision-Language Models, HOS 2.0 provides detailed scene descriptions, allowing the algorithm to understand the surrounding environment and driving context deeply. This enables the system to make informed and context-aware decisions.
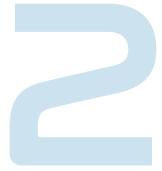
Human-Like Driving
The integration of “Thinking Fast and Slow” principles introduces dual decision-making systems
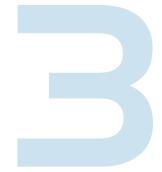
Elimination of Labeled Data
AV2.0 employs self-supervised learning, allowing the system to learn driving skills from raw, unlabeled data. This eliminates the need for expensive, labor-intensive labeled datasets, significantly accelerating the development cycle.
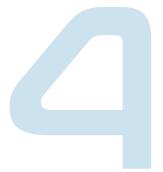
Vision-Only Perception
HOS 2.0 embraces simplicity by utilizing just 6–8 cameras for perception. Advanced algorithms enhance the system’s ability to make precise decisions, eliminating the need for complex and costly multi-sensor setups. This streamlined approach not only ensures high performance but also significantly reduces implementation costs.
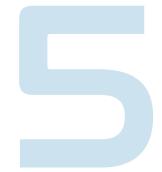
Drive Anywhere for Freedom
Unlike systems reliant on HD maps and pre-trained area , HOS 2.0 supports mapless autonomy. This capability allows seamless deployment in new geographies, leveraging data-driven adaptations to expand operational coverage effortlessly.
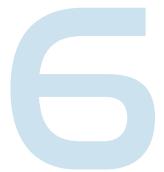
Vehicle Agnosticism
AV2.0 is designed to operate across various vehicle types, from heavy duty trucks to delivery vans. Innovations on one vehicle type directly benefit others, creating a versatile and adaptable autonomous driving solution.
This revolutionary framework sets a new standard for autonomous driving, combining advanced AI with practical flexibility to enable safer, smarter, and more human-like vehicle operation in any environment.
Hardware
The Best Eyes for Autonomous Vehicles
Advanced Computing Power
This revolutionary framework sets a new standard for autonomous driving, combining advanced AI with practical flexibility to enable safer, smarter, and more human-like vehicle operation in any environment.
Image capture is crucial for autonomous vehicles. We install six cameras on small to medium-sized vehicles and eight cameras on larger vehicles like container trucks. Our automotive-grade cameras feature a dynamic range of 120 dB, enabling them to capture scenes with significant differences in brightness without crushing shadows or overexposing highlights. The cameras are housed in anti-reflective and anti-condensation enclosures to ensure optimal performance in various conditions.
Our next-generation compute units are designed to support large-scale product deployments. We utilize NVIDIA AGX Orin or Drive Orin automotive SoCs to significantly improve power efficiency, reduce package size, and lower module costs. This approach simplifies our technology stack, enhancing performance and scalability while reducing the overall cost of our autonomous vehicle system.
Hardware
This revolutionary framework sets a new standard for autonomous driving, combining advanced AI with practical flexibility to enable safer, smarter, and more human-like vehicle operation in any environment.
The Best Eyes for Autonomous Vehicles
Image capture is crucial for autonomous vehicles. We install six cameras on small to medium-sized vehicles and eight cameras on larger vehicles like container trucks. Our automotive-grade cameras feature a dynamic range of 120 dB, enabling them to capture scenes with significant differences in brightness without crushing shadows or overexposing highlights. The cameras are housed in anti-reflective and anti-condensation enclosures to ensure optimal performance in various conditions.
Advanced Computing Power
Our next-generation compute units are designed to support large-scale product deployments. We utilize NVIDIA AGX Orin or Drive Orin automotive SoCs to significantly improve power efficiency, reduce package size, and lower module costs. This approach simplifies our technology stack, enhancing performance and scalability while reducing the overall cost of our autonomous vehicle system.
Drive-By-Wire System
Power Steering
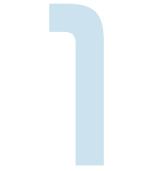
Maintains control of the steering wheel.
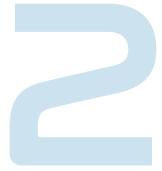
Simple and quick to install.
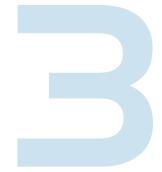
Removable if necessary.
Gas/Brake Control
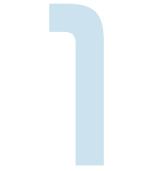
Adds to the existing brake system without altering it, keeping it fully operable by human drivers when necessary.
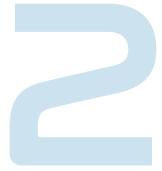
Enables brake operation by our software but can also be triggered by the human driver.
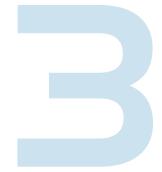
Prioritizes human input when the gas or brake pedal is pressed.
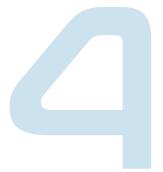
Can be disengaged at any time by the in-car driver or remotely.
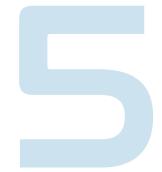
Compatible with almost all sedans, vans, mini-vans, trucks, and container trucks.
Safety
Our drive-by-wire system is fully redundant, consisting of two nearly identical components that continuously operate in parallel, monitoring themselves and each other. A malfunction in one component cannot result in a complete system failure; the other component ensures the brake remains operable by the driver. Any faults are promptly detected and communicated to the driver through audio signals or warning lights, ensuring maximum safety at all times.
Training with Generative AI
Generative AI World Model
We recognize that algorithms require extensive training on vast amounts of data across diverse scenarios. To facilitate this, we employ generative world models, which are revolutionizing deep learning alongside large language models (LLMs). These models simulate real-world environments, enabling AI to predict and interpret dynamic interactions within those environments. By forming general representations akin to human mental models, our AI enhances its decision-making capabilities and anticipates future events more effectively.
Training Model: Qubeley
Our training we named Qubeley, utilizes a combination of video, text, and action inputs to produce realistic driving videos with precise control over the ego-vehicle’s behavior and environmental features. Its multimodal nature allows Qubeley to generate videos from a variety of prompt modalities and their combinations, offering unparalleled flexibility and realism in simulations.
Key Contributions
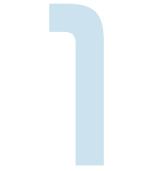
Universal Multimodal Planning Framework
We introduce an end-to-end multimodal planning framework via multi-target hydra-distillation. This allows our model to learn scalably from both rule-based planners and human drivers.
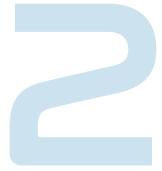
State-of-the-Art Performance
Our approach achieves state-of-the-art results based on simulation-based evaluation metrics within the Navsim environment.